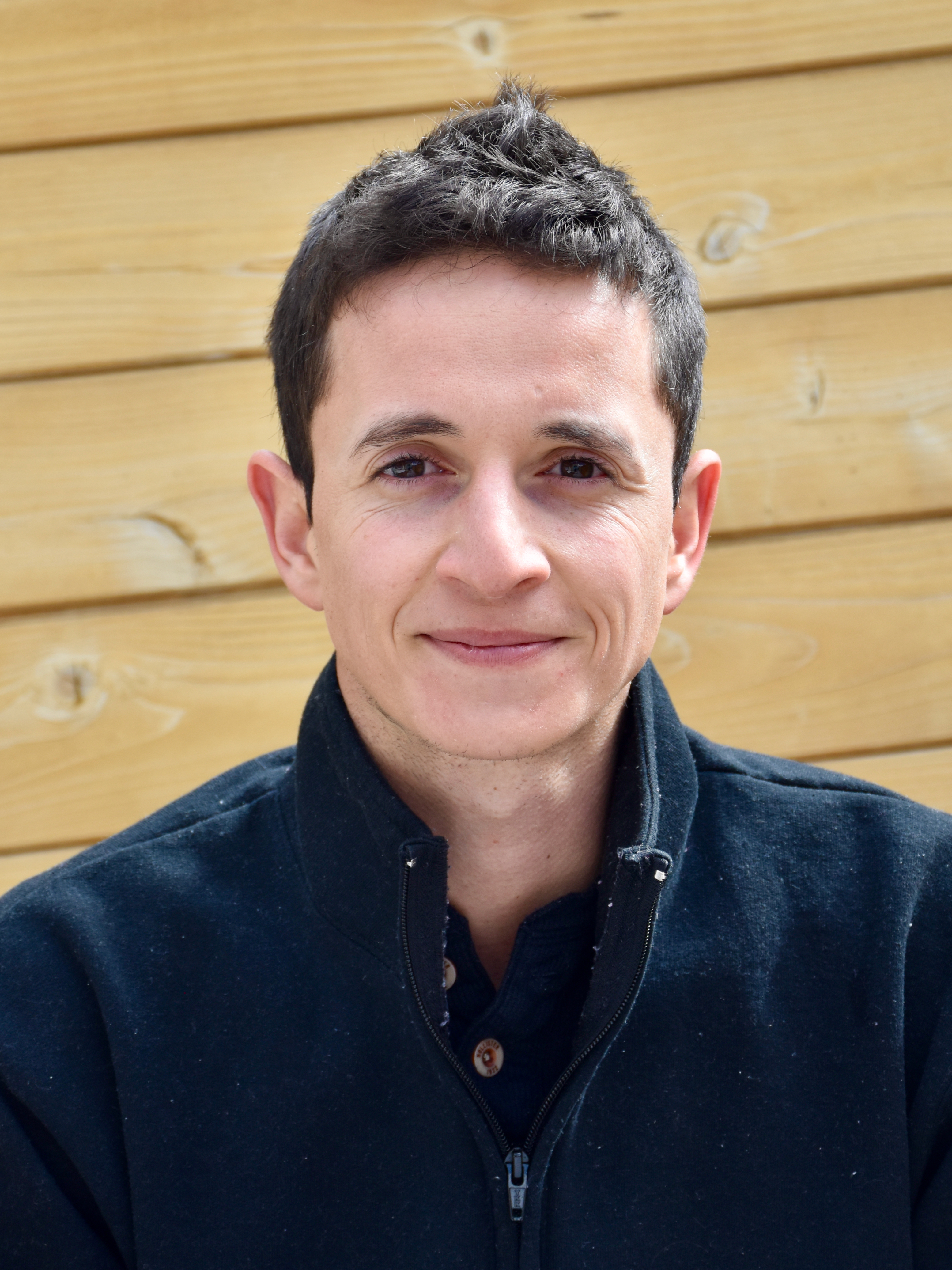
Currently
Senior Machine Learning Data Scientist & Technical Lead at Verily Life Sciences.
Technical Lead for a team of 4 building a suite of sensor-based digital measures and biomarkers for movement disorders. Our algorithms combine signal processing, statistics and deep learning to aggregate densely sampled time-series signals from wrist-worn wearable sensors into clinically relevant measures of motor impairment.
I always champion a problem-first approach: starting with the patient needs, we try off-the-shelf models and increase model complexity only where the performance benefit is clear. The model is a small part of the system; focus on simplicity, maintainability, clean data collection, rigorous validation, clean coding and testing.